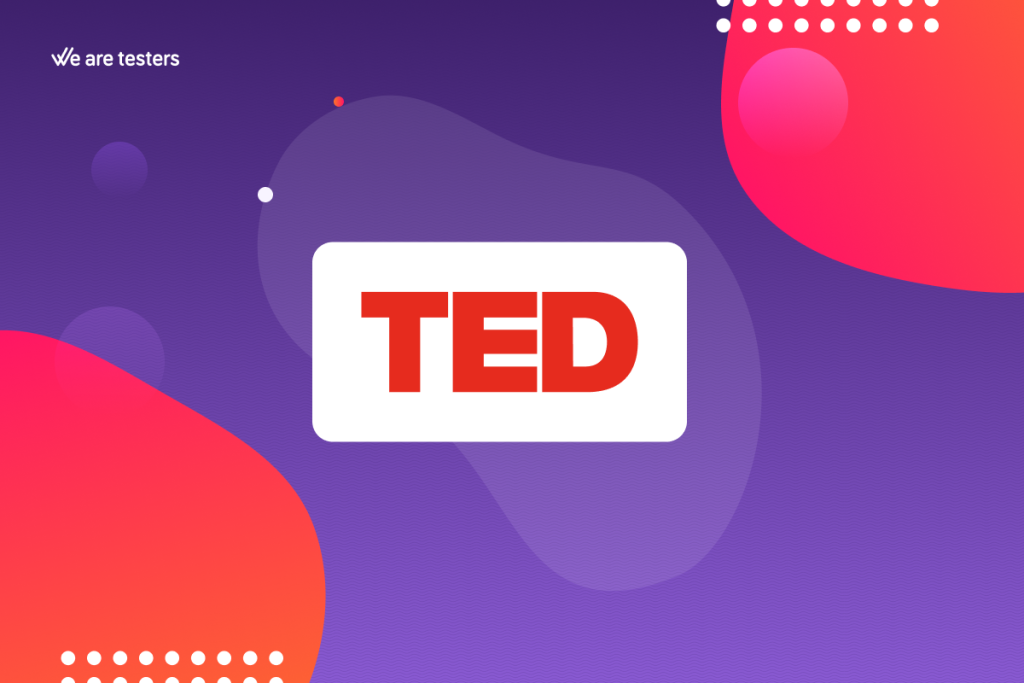
Artificial intelligence is here to stay in market research. The current state of technology allows many tasks to be carried out with the help of AI, and as it develops, it can be applied even more to make research easier, faster and of higher quality.
For that to happen, researchers need to learn how to use it, knowing where it adds value and where it does not. To shed light on the use of AI in practice, we have conducted a study that has allowed us to gather many interesting learnings.
Research on research
At We are testers we are researchers, so we don’t like to rely on data to make decisions. So, to understand the value of artificial intelligence in research, we wanted to do a «research on research» study by conducting the same research in three different ways:
Through artificial intelligence: seeing how far AI would take us (without human intervention other than prompts).
Through expert researchers. Doing the whole process through people without using artificial intelligence at any step.
Combining the use of experts and artificial intelligence.
In all cases, we evaluated the quality of the results and measured the time it took to complete the tasks. Our aim was to answer three questions:
- Can AI help us do research without losing quality?
- Can it help us to be faster and more efficient?
- How much more accurately?
To do the test we used a relatively simple and easy to solve study briefing via a quantitative online survey. To perform tasks with artificial intelligence we used solutions such as Chat GPT, DeepL hix.ai and Copilot; and we used them in three phases of the research: creation of the questionnaire, translation of the questionnaire and analysis of open questions.
Creating the questionnaire
The first step was to use the briefing to create a questionnaire using artificial intelligence. We tried different prompts that we polished and combined to see what we could come up with. At first, the results seemed spectacular. In a matter of minutes we had a questionnaire that at first glance answered the information objectives. The questions were correct and when the question involved composing lists of options – lists of brands or lists of the main means of finding out about the category – the AI created accurate lists for us. The rating scales were well constructed. Looking at the questions one by one, there was nothing wrong.
On that draft, one of our senior researchers reviewed the questionnaire in detail and made three major improvements.
The artificial intelligence stuck to the literal questions and created a question for each point in the briefing, in the same order and without any further thought. In the review, the researcher interpreted the briefing more openly, used her experience to imagine what the client was looking for behind the literal list of objectives and anticipated weaknesses in the briefing by filling in what would have been insufficient to make effective decisions.
Another important improvement was the reorganisation of the questionnaire. An expert researcher knows how to place the simpler questions at the beginning and leave the more complicated ones for when the respondent is more into the topic. And he or she also arranges the questions in such a way as to avoid having questions that might bias questions that will be asked later.
Our researcher also added text that enhanced the respondent’s experience by helping them better understand which block of the questionnaire they were in and valuing their participation. Motivating respondents and helping them to respond is key to getting quality responses.
While all this was going on, another researcher in our team conducted the questionnaire without the help of artificial intelligence. This way provided an even higher quality questionnaire. By starting from scratch, since the AI basis did not influence the researcher’s judgement, she was able to be more creative to go further in adding value. By contrast, it took her 60 minutes to complete the questionnaire, compared to the 40 minutes it took using the AI and revising her questionnaire. The 20-minute gain did not seem sufficient to compensate for the loss of quality in the questionnaire, a component that has a major influence on the quality of the results.
However, there were aspects in which the contribution of artificial intelligence did seem relevant. For example, in the study to be carried out, a list of brands and a list of media had to be compiled in order to find out about the product category. It took the researcher a relevant amount of time to search for information, while the AI composed the lists in a few seconds.
This is why we believe that the optimum lies in the combination of human work and artificial intelligence. The researcher is nowadays better at creating a well-structured and more valuable questionnaire. And at the same time, he can save time by using artificial intelligence to compose specific parts of the questionnaire, such as those involving contextual knowledge of the market and the category.
Translation of the questionnaire
The second task for which we tested the use of artificial intelligence was the translation of the questionnaire. We tested different machine translation solutions while in parallel sending our questionnaire to an external translation company. The result was that the agency’s translation and that of one of the AI solutions were virtually identical, but the use of the translation agency added cost and delayed the start of fieldwork by two days. With equivalent quality and virtually zero cost, we found the use of artificial intelligence in this field to be unassailable.
Analysis of open-ended questions
The third use case for artificial intelligence was the analysis of open-ended questions. This is a time-consuming task for researchers. Its analysis requires reading all the answers to get an idea of the main themes. It is even more time-consuming if you want to code the answers to obtain numerical results. In our case, an expert researcher spent 45 minutes to summarise the findings for each open-ended question (without coding). Using artificial intelligence we obtained a draft in seconds. This draft was tested by one of our researchers who certified its quality by reviewing a percentage of the respondents’ answers. This task, which we believe is important to validate that there is no failure of the AI to understand the answers, took 15 minutes per open-ended question.
If the quality is equivalent and 30 minutes per open-ended question is gained, we find the incorporation of artificial intelligence very useful for two reasons.
- Efficiency. Depending on the number of open questions in the questionnaire, several hours can be saved, which is relevant, especially in studies that require speed. In addition, manual work is reduced, which is generally incorporated into the price of the study.
- Increased value. Open-ended questions provide a great deal of value in research, yet they are used to a very limited extent in quantitative questionnaires. And that is because they are a lot of manual work …… So far. Thanks to artificial intelligence we can now use open-ended questions more frequently and access deeper information that was not accessible in practice before.
Conclusions
Looking at the results in perspective, we come to the following conclusions:
- Artificial intelligence is useful. It saves time and resources, allowing customers to get more out of their budget, make decisions faster and stay ahead of their competitors. It also opens the door to higher quality research through the possibility of using more open-ended questions in questionnaires. The contribution that AI makes to research is positive.
- AI does not replace researchers. There are tasks where the researcher is currently superior, such as research design, structuring the quality questionnaire and designing the respondent experience. Therefore, the researcher must retain control of the study and supervise the work of artificial intelligence where it is worthwhile to use it.
- Have a clear view on where AI adds value (and where it does not). It is important to experiment to understand where it brings benefits. And at the same time we must be demanding to continue to reserve those tasks where it does not yet provide the most value to humans.
- AI improves the researcher experience. Using AI to automate the most tedious tasks – such as analysing open-ended questions – improves the researcher experience and thus makes the profession more interesting. This helps research attract better professionals, which is good for the industry and for clients of research services.
Artificial intelligence at We are testers
We are testers’ research platform pioneered the introduction of artificial intelligence for the analysis of open questions in 2023. This functionality is available not only in online surveys, but also in online communities.
In light of the study results, we have introduced automatic translation of questionnaires in our development roadmap. This new functionality will be available during 2024.
In addition, we are already working on a questionnaire assistant that uses AI to help compose the questionnaire faster. This new functionality is planned for 2025.
On the We are testers development roadmap there are many other technological improvements such as the introduction of neuromarketing techniques – eyetracking and facial coding – which will be available in the coming months.
Follow us on LinkedIn or subscribe to get the latest news before anyone else. And if you miss anything, please contact us to find out more about the availability of any technology on our platform.
Update date 30 July, 2024